Jan Schindler
Senior Researcher - Remote Sensing and Data Scientist
Informatics
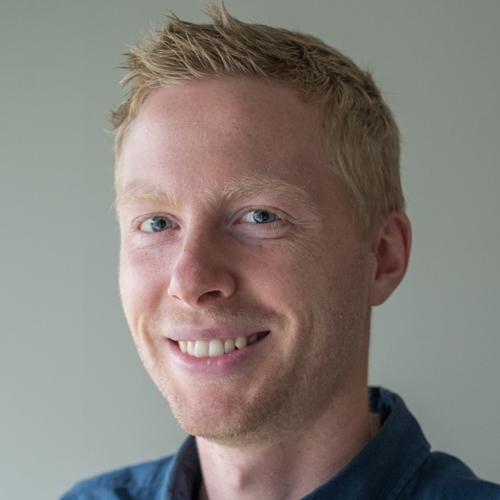
Location
Wellington
Research interests
- Natural processes and human influences on the Earth's surface and in its atmosphere by working across disciplines
- Studying Atmosphere-Land-Interactions by means of various remote sensing techniques
- Remote sensing, geospatial analysis and algorithms
- Geospatial modelling and interpolation
- Air pollution and short-lived atmospheric trace gases
- 3D point cloud analysis
- Machine learning methods for remote sensing
- Deep Learning using Convolutional Neural Networks
Research projects (selected)
- LiDAR Partnership Project between Hawke’s Bay Regional Council (HBRC) and Manaaki Whenua Landcare Research. Science lead of the vegetation mapping project: https://www.hbrc.govt.nz/services/maps-and-gis/lidar/
- Bridging the gap between remote sensing and tree modelling with data science - Science Leader - MBIE/SDSC Catalyst funded New Zealand-Singapore Data Science research programme lead by Manaaki Whenua - Landcare Research, project partners: Institute of High Performance Computing A*STAR, Nanyang Technological University, Scion, University of Canterbury, Victoria University of Wellington; more info: https://www.landcareresearch.co.nz/nzsg-datascience
- Advanced remote sensing of Aotearoa for next generation land cover mapping - Researcher - MBIE Endeavour funded project lead by Manaaki Whenua - Landcare Research
- Envirolink Tools project: A Collaborative Satellite Data Workspace for Regional Councils - Researcher - MBIE funded project lead by GNS Science, project partners: University of Auckland, Indufor, Xerra, Manaaki Whenua - Landcare Research; more info: https://www.gns.cri.nz/Home/Our-Science/Environment-and-Climate/Groundwater/Research-Programmes/Envirolink-Tools-project
Conference talks (selected)
- Experiences developing an operational workflow for large-scale instance and semantic segmentation of remote sensing imagery using CNNs, New Zealand Research Software Engineering Conference (NZRSE), 2021, Wellington, New Zealand
- A hi-fidelity approach for raster to vector conversions, GeoComputation, 2019, Queenstown, New Zealand
- Joint use of space-borne SAR, optical imagery and air-borne LiDAR for improved mapping of forest structural types in New Zealand, GeoComputation, 2019, Queenstown, New Zealand
- Using airborne LiDAR to study the spatial distribution of tall trees in Greater Wellington, New Zealand, EGU General Assembly 2019, Vienna, Austria
- Mapping forest alliance groups of indigenous forest in New Zealand from Sentinel, PALSAR, and LiDAR, EGU General Assembly 2019, Vienna, Austria
- Mapping tall trees in Greater Wellington using LiDAR data and an open-source tool chain, Keynote Talk at Science Coding Conference, 2018, Rotorua, New Zealand
- Investigation of photon path length distributions for cloudy atmospheres from GOSAT measurements of the oxygen A-band, 6th GOSAT RA PI Meeting, 2014, Tsukuba, Japan & 7th GOSAT RA PI Meeting 2015, Pasadena, CA, USA
- Rain-induced emission pulses of NOx and HCHO from soils in African regions after dry spells as viewed by satellite sensors, EUMETSAT conference, Geneva, Switzerland & EGU General Assembly, Vienna, Austria
- The potential of cloud slicing to derive profile information from Nadir looking instruments, GEMS Science Meeting, 2013, Seoul, South Korea
- Nördlinger Ries campaign on soil emissions: DOAS measurements of NO2 and HCHO in an agricultural region, EGU General Assembly, Vienna, Austria
Publications
Schindler, M., & Schindler, J. (2025). Understanding street tree inequities: The interrelation of urban layout and socio-economics. Urban Forestry & Urban Greening. https://doi.org/10.1016/j.ufug.2025.128765
Z. Sun, B. Xue, M. Zhang and J. Schindler, "YOLO-ITC: A New YOLO Method for Instance Segmentation of Individual Tree Crowns," in IEEE Transactions on Emerging Topics in Computational Intelligence, http://dx.doi.org/doi: 10.1109/TETCI.2025.3543833
Bleeker, L., Gonzalez, S., Wilson, A., Han, P., West, S., Scoggins, C., Shepherd, J., Schindler, J., Richardson, S., Fergus, A., Mason, E., Morgenroth, J., Xu, V., Jayathunga, S., & Camarretta, N. (2024). Remote Sensing to Measure Carbon Stock Changes. Report prepared for Ministry for Primary Industries (Agreement Number: C0034876). Interpine Group Ltd. Available online: https://www.mpi.govt.nz/dmsdocument/66294-Remote-Sensing-to-Measure-Carbon-Stock-Changes
Schindler, J., Sun, Z., Xue, B. and Zhang, M. (2024) Efficient Tree Mapping through Deep Distance Transform (DDT) Learning. Preprint. October 08, 2024. Available at SSRN: http://dx.doi.org/10.2139/ssrn.4979539
Sun, Z., Xue, B., Zhang, M., & Schindler, J. (2024).
YOLOv8E: an efficient YOLOv8 method for instance segmentation of
individual tree crowns in Wellington City, New Zealand. Journal of the
Royal Society of New Zealand, 1–26.
https://doi.org/10.1080/03036758.2024.2424802
Pronger J, Price R and Schindler, J. (2024) "Estimating carbon emissions from peatland fires at Kaimaumau–Motutangi and Awarua wetlands" Manaaki Whenua - Landcare Research contract report LC4468 prepared for Department of Conservation, 36p. Available online at: https://www.doc.govt.nz/globalassets/documents/conservation/land-and-freshwater/wetlands/estimating-carbon-emissions-from-peatland-fires.pdf
B. Martin, J. D. Shepherd, N. Mason and J. Schindler, (2024) "Improving New
Zealand’s vegetation mapping using weakly supervised deep learning," International Conference on Machine Intelligence for GeoAnalytics and Remote Sensing (MIGARS), Wellington, New Zealand, 2024, pp. 1-3, http://dx.doi.org/10.1109/MIGARS61408.2024.10544907
B. Xu, B. Xue, J. Schindler and M. Zhang, (2024) "Ensemble learning based on neural networks for tree image segmentation," International Conference on Machine Intelligence for GeoAnalytics and Remote Sensing (MIGARS), Wellington, New Zealand, 2024, pp. 1-3, http://dx.doi.org/10.1109/MIGARS61408.2024.10544793
Martin, B., Schindler, J., McMillan, A., & Burge, O. (2024) Mapping difficult wetlands. Manaaki Whenua - Landcare Research contract report LC4468 prepared for Ministry for the Environment, 62p. Available online at: https://datastore.landcareresearch.co.nz/dataset/wetland-mapping-resources
Schindler, J. (2024). LiDAR-derived vegetation layers in Hawke's Bay. Manaaki Whenua – Landcare Research contract report LC4470 prepared for Hawke’s Bay Regional Council, 40p. Available online at: https://www.hbrc.govt.nz/our-documents/reports-search/details/16437
Gobeawan, L.; Liu, X.; Lim, C.; Raghavan, V.; Chattoraj, J.; Schindler, J. and Yang, F. (2024). TreeSpecies-PC2DT: Automated Tree Species Modeling from Point Clouds to Digital Twins. In Proceedings of the 19th International Joint Conference on Computer Vision, Imaging and Computer Graphics Theory and Applications - GRAPP; SciTePress, pages 81-91. https://www.scitepress.org/publishedPapers/2024/123897/pdf/index.html
Z. Sun, B. Xue, M. Zhang and J. Schindler, (2023) "An Improved Mask R-CNN for Instance Segmentation of Tree Crowns in Aerial Imagery," 38th International Conference on Image and Vision Computing New Zealand (IVCNZ), Palmerston North, New Zealand, 2023, pp. 1-6. https://dx.doi.org/10.1109/IVCNZ61134.2023.10343827
Zhao, H., Morgenroth, J., Pearse, G., & Schindler, J.
(2023). A Systematic Review of Individual Tree Crown Detection and
Delineation with Convolutional Neural Networks (CNN). Current Forestry
Reports, 1-22. https://doi.org/10.1007/s40725-023-00184-3
Martin, B., Belliss, S., Pairman, D., Soliman, T., Schindler, J. and Amies, A., (2022). Quantifying the historical evolution of green space in New Zealand’s cities. Manaaki Whenua – Landcare Research contract report LC5034 prepared for Parliamentary Commissioner for the Environment, 59p. Available online at: https://pce.parliament.nz/media/j1gnsvra/manaaki-whenua-quantifying-the-historical-evolution-of-green-space-in-new-zealands-cities.pdf
Martin, B., Belliss, S., Pairman, D., Soliman, T., Schindler, J. and Amies, A., (2022). Quantifying the historical evolution of green space in New Zealand’s cities. Extension: Measuring urban green space and vegetation from infrared imagery. Manaaki Whenua – Landcare Research contract report LC4159 prepared for Parliamentary Commissioner for the Environment, 42p. Available online at: https://pce.parliament.nz/media/jh1lzghv/manaaki-whenua-quantifying-the-historical-evolution-of-green-space-in-new-zealands-cities-measuring-urban-green-space-and-vegetation-from-infrared-imagery.pdf
Spiekermann, R. I., van Zadelhoff, F., Schindler, J., Smith, H., Phillips, C., & Schwarz, M. (2023). Comparing physical and statistical landslide susceptibility models at the scale of individual trees. Geomorphology, 440, 108870. https://doi.org/10.1016/j.geomorph.2023.108870
Fu, W., Xue, B., Zhang, M. and Schindler, J. (2023) February. Evolving U-Nets Using Genetic Programming for Tree Crown Segmentation. In Image and Vision Computing: 37th International Conference, IVCNZ 2022, Auckland, New Zealand, November 24–25, 2022, Revised Selected Papers (pp. 188-201). Cham: Springer Nature Switzerland. https://doi.org/10.1007/978-3-031-25825-1_14
Xu, B., Bi, Y., Xue, B., Schindler, J., Martin, B. and Zhang, M., (2022) December. Automatically Designing U-Nets Using A Genetic Algorithm for Tree Image Segmentation. In 2022 IEEE Symposium Series on Computational Intelligence (SSCI) (pp. 626-633). IEEE. https://dx.doi.org/10.1109/SSCI51031.2022.10022182
Jolly B, Dymond JR, Shepherd JD, Greene T, Schindler J. (2022) Detection of Southern Beech Heavy Flowering Using Sentinel-2 Imagery. Remote Sensing. 14(7):1573.
https://doi.org/10.3390/rs14071573
North, H., Amies, A., Dymond, J., Belliss, S., Pairman, D., Drewry, J., Schindler, J., and Shepherd, J.: Mapping bare ground in New Zealand hill-country agriculture and forestry for soil erosion risk assessment: An automated satellite remote-sensing method, Journal of Environmental Management, 301, 113812, https://doi.org/10.1016/j.jenvman.2021.113812, 2022.
Schindler, J., Dymond, J. R., Wiser, S. K., and Shepherd, J. D. (2021) Method for national mapping spatial extent of southern beech forest using temporal spectral signatures, International Journal of Applied Earth Observation and Geoinformation, 102, 102408, https://doi.org/10.1016/j.jag.2021.102408
Shepherd, J.D.; Schindler, J.; Dymond, J.R. (2020) Automated Mosaicking of Sentinel-2 Satellite Imagery. Remote Sens., 12, 3680. https://doi.org/10.3390/rs12223680
Amies, A., Belliss, S., North, H., Pairman, D., Dymond, J., Schindler,
J., Shepherd, J. and Drewry, J., 2020. Classification of Winter Land
Cover in New Zealand Hill Country for Risky Practice Identification. In IGARSS 2020-2020 IEEE International Geoscience and Remote Sensing Symposium (pp. 2751-2754). IEEE. https://dx.doi.org/10.1109/IGARSS39084.2020.9323159
Belliss, S.E.; Pairman, D.; Dymond, J.; Amies, A.; Zoerner, J.; Shepherd, J.; Drewry, J.; North, H. (2019) Identification of high-risk agricultural activities: national mapping of the location, scale and extent of winter forage cropping and intensive grazing on hill country land. Landcare Research contract report LC3469 prepared for Ministry for the Environment, 61p. Available online at: https://environment.govt.nz/assets/Publications/Files/identification-of-high-risk-agricultural-activities-on-hill-country-land.pdf
Dymond, J.R.; Zörner, J.; Shepherd, J.D.; Wiser, S.K.; Pairman, D.; Sabetizade, M. (2019) Mapping Physiognomic Types of Indigenous Forest using Space-Borne SAR, Optical Imagery and Air-borne LiDAR, Remote Sens. 2019, 11(16), 1911; https://doi.org/10.3390/rs11161911
Etherington, T.R., Jolly, B., Zörner,
J. and Spencer, N., 2019. A research institution framework for
publishing open code to enable reproducible science. PeerJ Preprints, 7, p.e27762v1. https://peerj.com/preprints/27762.pdf
Zörner, J.; Dymond, J.R.; Wiser, S.K.; Shepherd, J.D. and Jolly, B. (2018) LiDAR-Based Regional Inventory of Tall Trees—Wellington, New Zealand. Forests, 9, 702, https://doi.org/10.3390/f9110702
Zörner, J.; Dymond, J.; Shepherd, J. and Jolly, B. (2018) PyCrown - Fast raster-based individual tree segmentation for LiDAR data. Landcare Research NZ Ltd., https://doi.org/10.7931/M0SR-DN55
Zörner, J (2017) Remote sensing study of NOx emissions from soils using space- and ground-based DOAS instruments, Ph.D. thesis, Johannes Gutenberg University Mainz, Germany, http://doi.org/10.25358/openscience-4867
Zörner, J.; Penning de Vries, M.; Beirle, S.; Sihler, H.; Veres, P. R.; Williams, J. and Wagner, T. (2016) Multi-satellite sensor study on precipitation-induced emission pulses of NOx from soils in semi-arid ecosystems, Atmos. Chem. Phys., 16, 9457-9487, https://doi.org/10.5194/acp-16-9457-2016
Wagner, T.; Penning de Vries, M.; Beirle, S. and Zörner, J. (2013) Investigation of trace gas to aerosol relationships over biomass burning area using daily satellite observations. ESA Living Planet Symposium, Proceedings of the conference held on 9-13 September 2013 at Edinburgh in United Kingdom. ESA SP-722. 2-13, p.133 https://ftp.space.dtu.dk/pub/Ioana/papers/s482_5wagn.pdf
Verstraeten, W. W.; Boersma, K. F.; Zörner, J.; Allaart, M. A. F.; Bowman, K. W. and Worden, J. R. (2013) Validation of six years of TES tropospheric ozone retrievals with ozonesonde measurements: implications for spatial patterns and temporal stability in the bias, Atmos. Meas. Tech., 6, 1413-1423, https://doi.org/10.5194/amt-6-1413-2013
Zörner, J. (2012) Evaluating spaceborne tropospheric ozone time series with sonde data, M.Sc-thesis, Faculty of Bioscience-Engineering, KU Leuven, Leuven, Belgium, https://doi.org/10.5281/zenodo.1451944
Qualifications
Johannes Gutenberg
PhD Natural Sciences
2017
Leuven, Belgium
MSc Earth Observation
2012
Munich, Germany
BSc Geography
2006