Use of cameras and artificial intelligence to monitor wildlife
Image recognition software has the potential to automate the processing of photographs, making camera trapping much more time- and cost-effective. Al Glen and colleagues have used software developed for Australian animals and adapted it to identify species found in New Zealand. Using a machinelearning approach known as Deep Metric Learning, they ‘trained’ computer models to identify camera trap images of stoats, cats, hedgehogs, livestock, kiwi and other birds. The models were initially trained using a few hundred sample images of each species and achieved up to 75% accuracy with independent test data. With help from collaborators around New Zealand, Al’s team is compiling much larger numbers of sample images to improve the accuracy of species recognition. They aim to collect over 10,000 images of each species and anticipate that accuracy will reach well over 90%.
The list of species that the software will recognise is growing longer. Driven partly by the Predator Free 2050 objective, two high priorities are to train the software to identify rodents and possums, and to investigate whether artificial intelligence can reliably distinguish between rats and mice. Other species to be added include ferrets, rabbits, hares, pigs and dogs.
The software first identifies whether an animal is present in each image. This is challenging due to extreme variability in background and lighting conditions when monitoring wild animals. Sample images therefore include a wide variety of backgrounds (e.g. pasture, forest, tussock) and lighting conditions (e.g. bright light, low light, dappled shade).
If an animal is present, the software produces a copy of the image with a box drawn around the animal, a label (e.g. cat or stoat) and a confidence rating for the identification. The software can also identify more than one animal in the same image. The images are then sorted into folders according to species, and a spreadsheet is produced showing the species identified in each photograph.
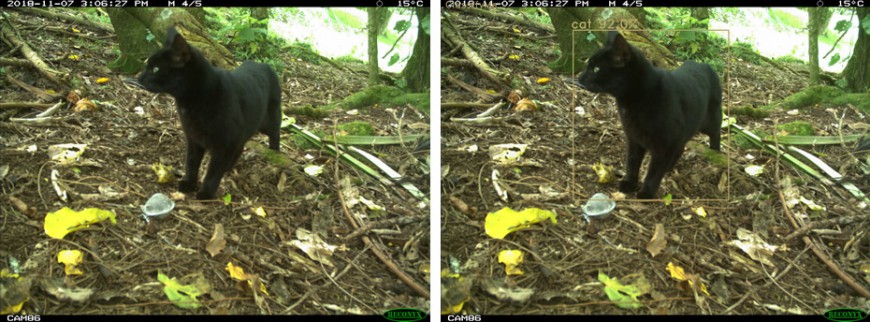
When the artificial intelligence software identifies an animal in a photograph, it draws a box around the animal, labels it by species and gives a confidence rating for the identification. This helps the user to check the accuracy of identifications. ‘Clean’ copies of each image are saved into folders according to species, and a spreadsheet is produced showing the species identified in each image.
Processing speed will vary depending on the computer used, the speed of the internet connection, and the size of the image files. In early trials with large numbers of photographs, processing speeds between 10 and 30 times faster than manual image processing have been achieved.
In collaboration with Groundtruth Ltd, Al and his colleagues also plan to develop a user interface to allow the image recognition software to be used by conservation organisations, community groups and researchers throughout New Zealand. The software will be made freely available for wildlife researchers and practitioners through Trap.NZ (www. trap.nz). Users will be able to upload their camera trap images and have animal species automatically identified and tagged by artificial intelligence.
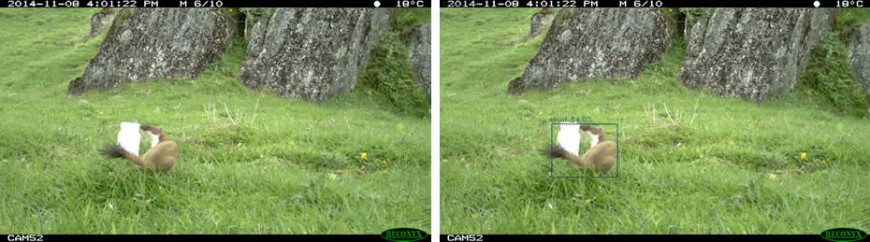
When the artificial intelligence software identifies an animal in a photograph, it draws a box around the animal, labels it by species and gives a confidence rating for the identification. This helps the user to check the accuracy of identifications. ‘Clean’ copies of each image are saved into folders according to species, and a spreadsheet is produced showing the species identified in each image.
A number of questions and approaches need further investigation. At what confidence rating should a species identification be considered reliable? For example, managers may use manual processing to check any images with a confidence rating below a certain threshold. Future work will also improve the software’s ability to identify animals in images with different backgrounds (e.g. grassland, shrubland, etc.).
With further development, artificial intelligence will make camera trapping achievable and cost-effective at the large scales required for Predator Free 2050.
This work was funded by MBIE under the Kiwi Rescue Endeavour Programme, and by PF2050 Products to Projects funding.
Key contacts
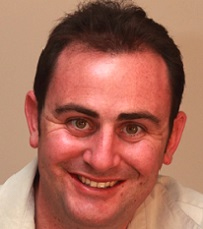